Monitoring confirmed cases of COVID-19 reveals particularly fast-spreading patterns. This kinetics is characteristic of all epidemiological dynamics. It is based on the fact that each case of contamination causes multiple others, through the mixing of population.
The aim of this article is to explain in a didactic way the dynamics of epidemiology.
From an exponential epidemiological spread…
At first glance, I(t) noting the number of cumulative confirmed cases at t (most often for a daily unit of time), the increasing number of confirmed cases can be approximated by the following relationship:
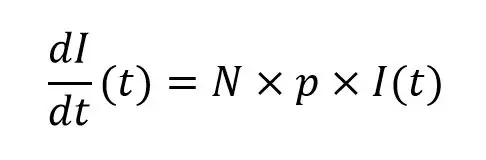
Noting a = N . p the infection rate, solving this equation leads to an exponential profile of the number of confirmed cases, similar to the profile observed during the active development phase of the pandemic:
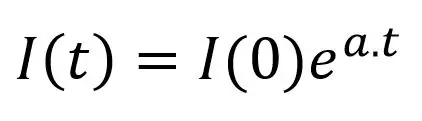
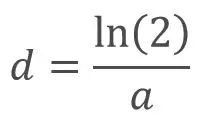
…to a logistic convergence
In practice, the growth of confirmed cases does not correspond to an exponential dynamic which does not take into account the regular decreasing of “susceptible” populations (i.e. the contaminable individuals).
Indeed, the average number of individuals encountered by an infected person at each unit of time must be reduced by the number of already infected populations, to account for the depletion of susceptible persons over time:
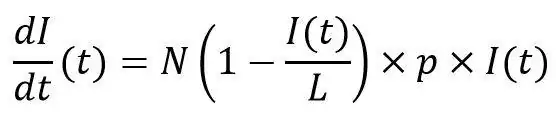
This differential equation, known as the Verhulst model, leads to the following solution:
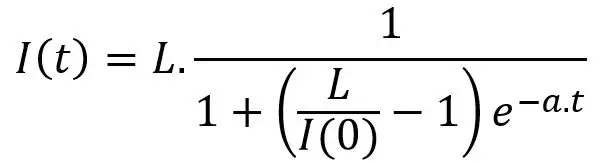
This function, most often referred to as the “logistic function”, induces profiles that are relatively faithful to those of the cumulative numbers of confirmed cases observed during a fully developed pandemic episode (e.g. the COVID-19 epidemic in China).
Some examples
The parameters of the Verhulst’s dynamics were calibrated on the Chinese data outside Hubei[1], from 01/22/2020 to 03/20/2020[2].
The objective function considered is the sum of the squared deviations between the observed and theoretical cumulative confirmed case.
The graph below illustrates the adjustment of the Verhulst’s dynamics on confirmed Chinese cases outside of Hubei.
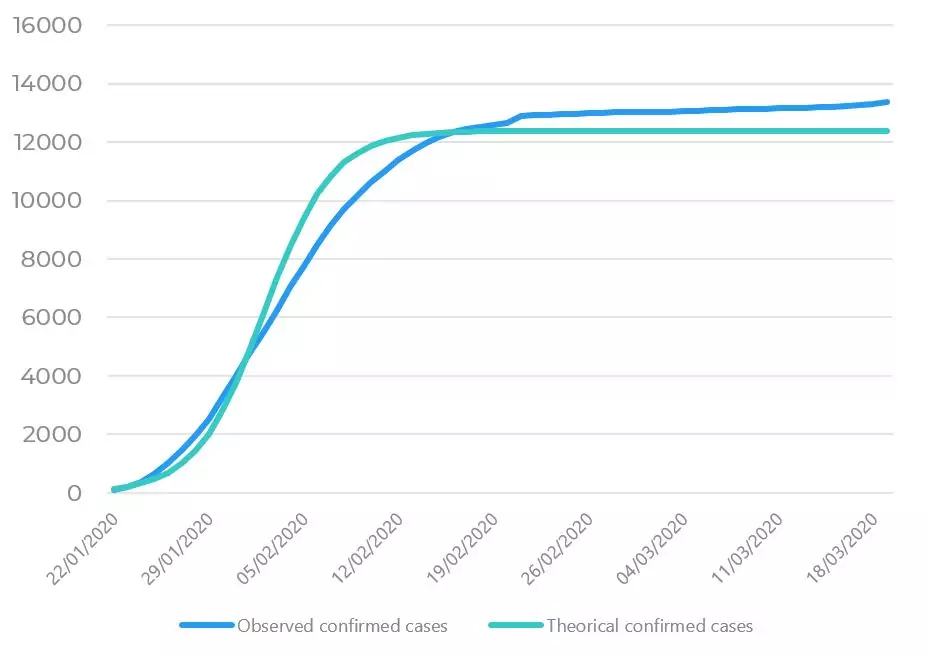
Figure 1: Cumulative observed and theoretical confirmed cases (China excluding Hubei)
The variation in the number of confirmed cases per unit of time (i.e. the quantities ) is increasing during the first stage of the pandemic then gradually decreasing. The function is convex at the beginning of the pandemic, then concave when an inflection point is reached. This epidemic peak, particularly monitored by the health authorities, is characterized by this pivotal mechanism.
The following graph provides a comparison of observed and theoretical confirmed cases on Chinese data (excluding Hubei).
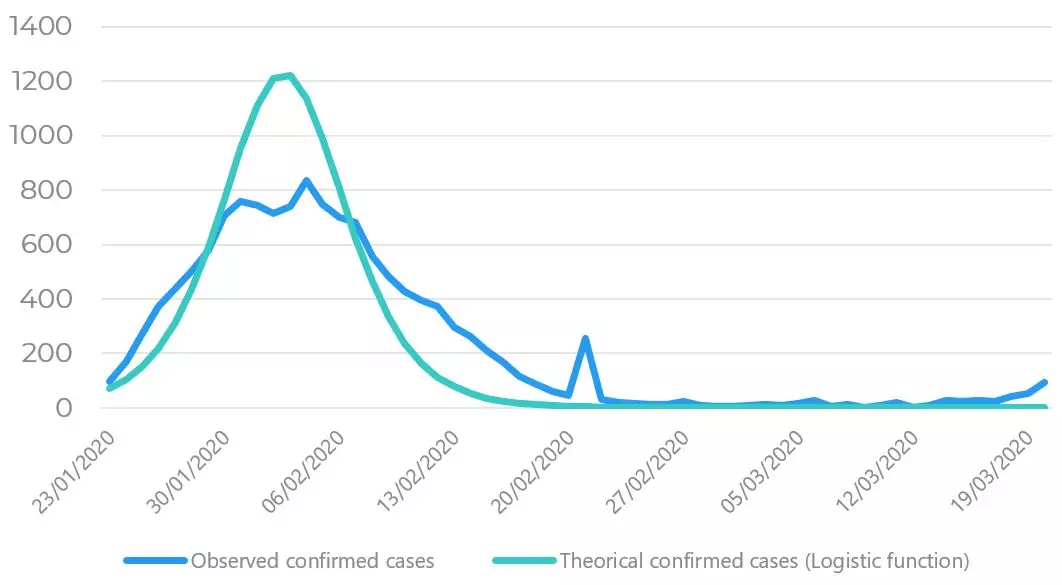
Figure 2: Observed and theoretical confirmed cases (China excluding Hubei)
The graphs above show an epidemic peak in China (excluding Hubei) around 02/04/2020 with a maximum daily variation of confirmed cases reaching 835 individuals.
Conclusion
The exponential and logistic dynamics explain the speed at which the epidemic is spreading. For all countries affected by the COVID-19 outbreak, the increasing number of cases during the first stage of the epidemic is quite consistent with an exponential profile. Nevertheless, the Verhulst’s dynamics is more appropriate for modeling the epidemic spread independently of the lockdowns, as it takes into account the depletion of susceptible individuals.
However, these simplified models cannot, as such, constitute efficient predictive tools to anticipate the medium-term development of the epidemic. To do so, dedicated epidemiological models should be used, as it allows the real-time dynamization of different population compartments and their respective interactions. This is the case of SIRD-type models (Susceptible – Infected – Recovered – Deaths), which will be the subject of a subsequent article.
By addactis® – April 2020
Laurent Devineau, Executive Partner
Marielle de La Salle, Head of Modeling Lab
Auriol Wabo, Consultant
Carolina Ramirez, Regional Head of Consulting
[1] Data concerning Hubei province around 02/12/2020 were not taken into account considering the change in counting scheme.
[2] Johns Hopkins University – https://github.com/CSSEGISandData/COVID-19